In the span of only a few months, artificial intelligence became the hottest topic in the tech industry and beyond. Its current poster child, ChatGPT, reached 100 million users just two months after it launched in November 2022, achieving a faster growth rate than any other consumer application in history.
However, what we’re witnessing today is merely the tail end of a long and fascinating journey that began decades ago. In this blog post, I chart the outlines of the long and often winding road that has led us to the current exponential rise of intelligent machines.
Early Beginnings in the 1950s
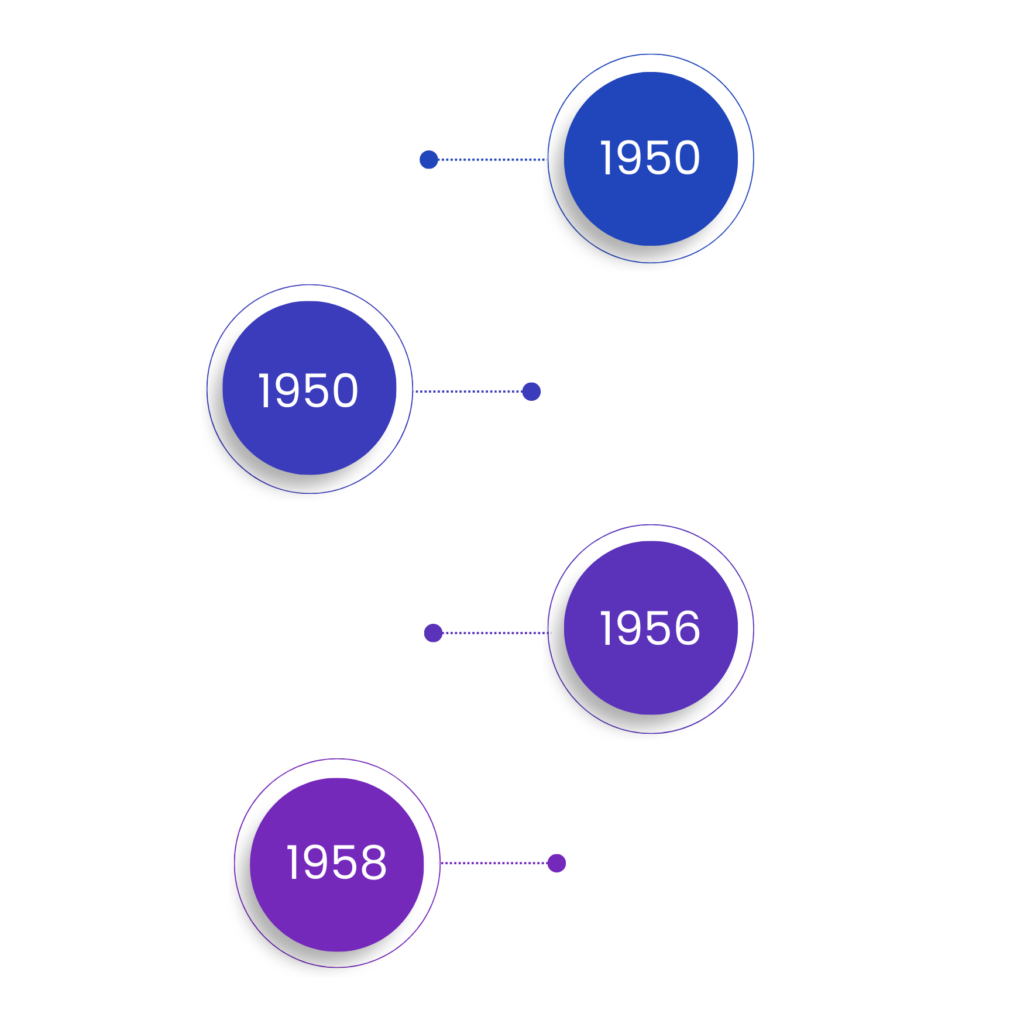
While philosophers, inventors, and everyday people alike have dreamt about intelligent machines for centuries, the real dawn of artificial intelligence can be traced back to the 1950s.
One of the first AI systems to capture the imagination of the public was Theseus, a remote-controlled maze-solving mouse built by Claude Shannon in 1950. Inspired by the ancient Greek myth of Theseus, who navigated a minotaur’s labyrinth using a thread to mark his path, Shannon’s electromechanical mouse had the ability to “remember” its path through the use of telephone relay switches.
That same year, Alan Turing came up with what would later become the now-famous Turing Test, which challenged machines to convince humans they were chatting with another human. This litmus test set the stage for evaluating whether machines could be considered “intelligent.”
In 1952, Arthur Samuel from IBM started working on a computer program for playing checkers. The program was demonstrated on television in 1956, and its later versions achieved good results despite the significant limitations of the hardware it ran on, the IBM 701.
Inspired by Arthur Samuel’s machine learning efforts and Donald Hebb’s model of brain cell interaction, Frank Rosenblatt created a simplified model of a biological neuron, called the perceptron, at the Cornell Aeronautical Laboratory. This model was then implemented in custom-built hardware designed for image recognition as the “Mark 1 perceptron”.
Pre Deep Learning Era
The 1960s marked a period of rapid innovation in the world of artificial intelligence. People were captivated by the possibilities of AI, as evidenced by the flurry of new programming languages, robots, research studies, and even movies that showcased the potential of intelligent machines.
In 1965, computer scientist Joseph Weizenbaum unveiled ELIZA, an interactive computer program that could hold a functional conversation in English. Although Weizenbaum’s intention was to show how superficial communication between AI and humans could be, he was surprised to find that many users attributed human-like qualities to ELIZA.
The nearest neighbor algorithm, developed in 1967, signaled the beginning of basic pattern recognition. This algorithm was initially used for mapping routes and became one of the earliest solutions to the traveling salesperson problem.
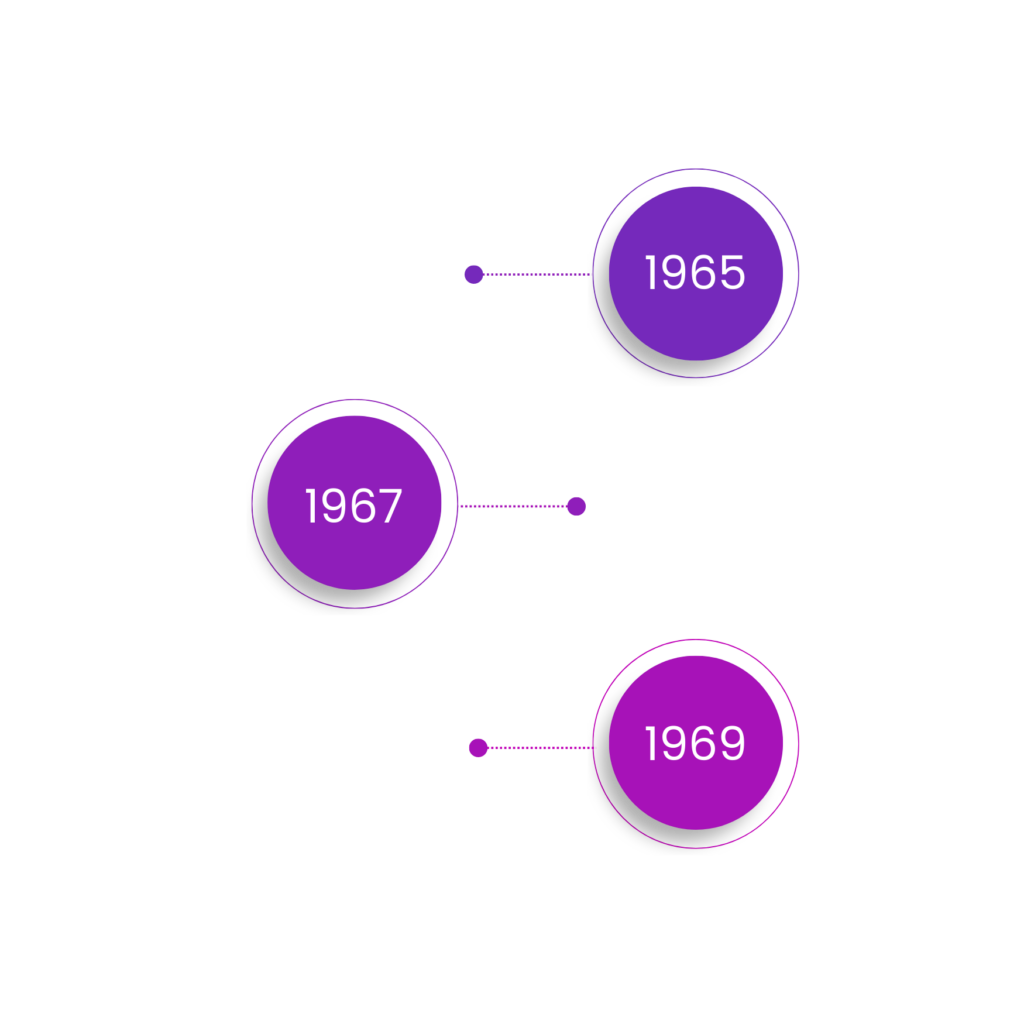
Another major breakthrough that arrived in the 1960s was the discovery of the multilayer artificial neuron network design. This discovery led to the development of backpropagation, which enables a network to adjust its hidden layers to adapt to new situations. Today, backpropagation is used to train deep neural networks, which give the name to the current era of artificial intelligence.
Deep Learning Era
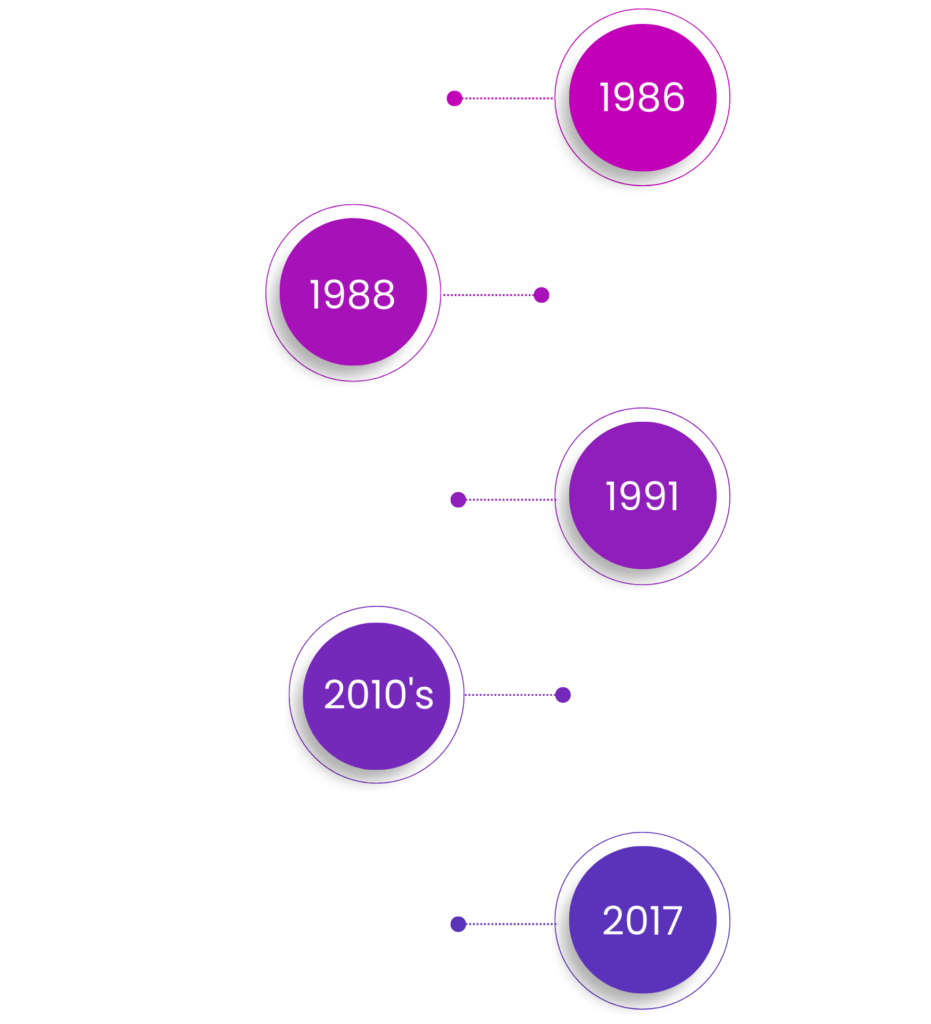
The term “Deep Learning” was first introduced by Rina Dechter to the machine learning community in 1986, and it has since become a game-changer for artificial intelligence, propelling it to new heights of capability and understanding.
Deep learning algorithms use multiple layers to progressively extract higher-level features from raw input. In image processing, for example, lower layers might identify edges, while higher layers could recognize more complex concepts like digits, letters, or faces.
In the early days of deep learning, researchers like Wei Zhang and his colleagues were already pushing the boundaries of what AI could achieve. In 1988, they applied the backpropagation algorithm to a convolutional neural network for alphabet recognition. In 1991, they modified their model to apply deep learning techniques to medical image object segmentation and later breast cancer detection.
These early applications of deep learning algorithms showcased the incredible potential of AI to revolutionize industries and improve our daily lives. By the late 2010s, companies began developing large-scale machine learning models that required 10 to 100 times more compute power for training.
The landscape of AI was forever changed with the introduction of the Transformer model in 2017 by Ashish Vaswani and his team. Their groundbreaking paper, “Attention Is All You Need,” showcased a deep learning model that used self-attention to weigh the significance of each part of the input data.
Transformers quickly became the go-to model for natural language processing (NLP) tasks, and they now serve as the foundation for many modern large language models like OpenAI’s ChatGPT or Google’s BERT.
The Months When AI Went Mainstream
After decades of major breakthroughs occurring at a normal annual to bi-annual rate despite being mostly invisible to the general public, we’re now witnessing an incredible surge in the accessibility and application of AI across industries and use cases. November 30, 2022 was the date ChatGPT was launched and shook many technology enthusiast to the core. Since the turn of 2023, the industry has seen an absolute onslaught of announcements and innovations totaling in the double digits per week at times. From AI art generators like Stable Diffusion, Dall-E, and MidJourney to RunWay, Nvidia, Microsoft, Google, Amazon, and hundreds of new AI focused companies making waves such as Pinecone.
March 2023 will most likely go down the history as the month when AI news exploded, as technology giants and innovative startups alike pushed the boundaries of AI applications.
Stanford University released Alpaca 7B, a state-of-the-art AI model (based on Meta’s LLaMA 7B model) that anyone can tinker with thanks to its open-source license. Meanwhile, Google announced Med-PaLM 2, a new medical LLM designed to revolutionize healthcare research and diagnostics. Not to be outdone, OpenAI released the much-anticipated GPT-4, setting a new benchmark for natural language processing and generation.
In a bid to enhance productivity and creativity in the workplace, Google added generative AI capabilities to its workspaces, while Midjourney released V5 for all paid users, offering even more advanced text-to-image generation capabilities. Microsoft cemented its leading role by releasing an AI Copilot for the 365 suite, empowering users with AI-driven assistance and insights across a range of applications.
As the month progressed, the momentum showed no signs of slowing down. Google announced a waitlist for Bard, an innovative generative AI platform, while Adobe unveiled Firefly, a cutting-edge AI-powered design tool. GitHub introduced GitHub Copilot X, a powerful AI-driven coding assistant, and Opera unveiled generative AI tools for its web browser.
Given the mind-blowing rate at which new AI developments and product launches are now happening, it comes as no surprise that the interest in AI, as evidenced by Google Trends data, is exploding. With AI-based tools now readily available and increasingly deeply embedded in a wide array of sectors, it’s clear that AI has become a mainstream phenomenon.
Democratization of AI Technologies Continues
However, the fact that AI-related announcements have moved from academic journals to mainstream media headlines is one consequence of the exponential rise of intelligent machines. Another equally important consequence is the democratization of AI technologies, which has allowed even hobbyists to access powerful tools and resources that were once reserved for only a handful of experts.
A variety of tools and libraries have emerged, significantly lowering the barriers to entry and enabling a broader range of participants to contribute to the AI revolution. For example, Hugging Face’s Transformers library and LangChain have made it easier than ever to implement natural language processing applications and combine large language models with other sources of computation or knowledge.
Moreover, open-source language models like the aforementioned Stanford’s Alpaca , EleutherAI’s GPT-J, or StableVicuna make it possible for anyone to innovate without the shackles that come with commercial products like ChatGPT.
Thanks to them, the world of AI has become more inclusive and accessible. A prime example of this is the Alpaca.cpp project, which allows users to run a fast ChatGPT-like model locally on their devices, even on relatively modest hardware, such as an M1 MacBook Air.
As the exponential growth and democratization of AI continue, one thing is clear, AI is here to shape the future and play a major role in society, our work and our entertainment.
Conclusion
From the early beginnings of AI in the 1950s to the current era of deep learning and large language models, the evolution of intelligent machines has been a fascinating journey. The democratization of AI has opened doors to groundbreaking discoveries and applications that will continue to transform industries and improve our daily lives. Ultimately, the AI evolution is a testament to human ingenuity and the relentless pursuit of knowledge. We just need to remember that with great knowledge comes great responsibility. If you are interested in learning how AI could be implemented today to increase productivity and augment your enterprise, please reach out to our team at Problem Solutions to continue the conversation.